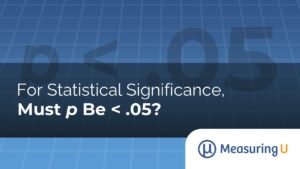
For Statistical Significance, Must p Be < .05?
If you know even just a little about statistics, you know that the value .05 is special. When the p-value obtained from conducting a statistical test falls below .05, it typically gets a special designation we call statistically significant. This is the conventional threshold for publishing findings in academic journals, and consequently, it is ascribed